Publications
- Beam Prediction
- Blockage Prediction
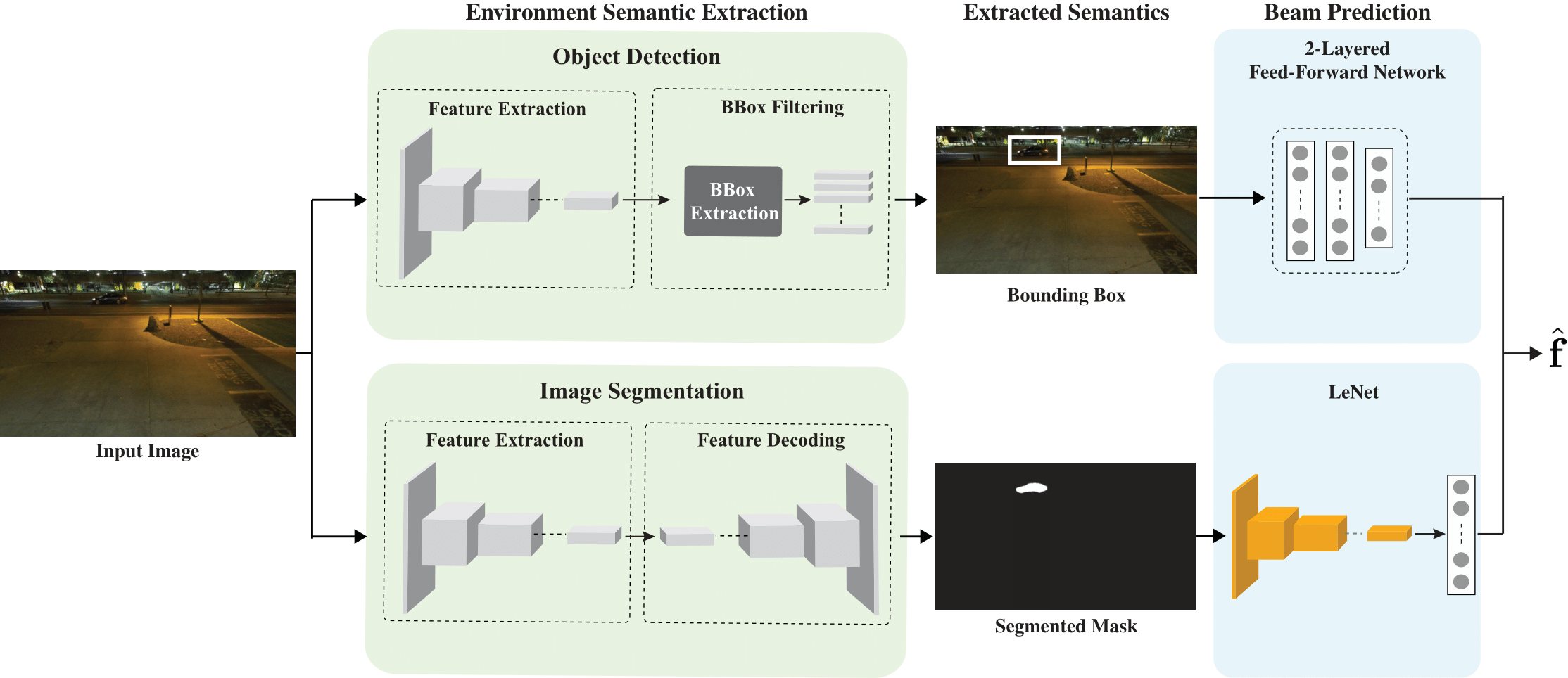
Environment Semantic Aided Communication: A Real World Demonstration for Beam Prediction
Millimeter-wave (mmWave) and terahertz (THz) communication systems adopt large antenna arrays to ensure adequate receive signal power. However, adjusting the narrow beams of these antenna arrays typically incurs high beam training overhead that scales with the number of antennas. Recently proposed vision-aided beam prediction solutions, which utilize raw RGB images captured at the basestation to predict the optimal beams, have shown initial promising results. However, they still have a considerable computational complexity, limiting their adoption in the real world. To address these challenges, ... read more
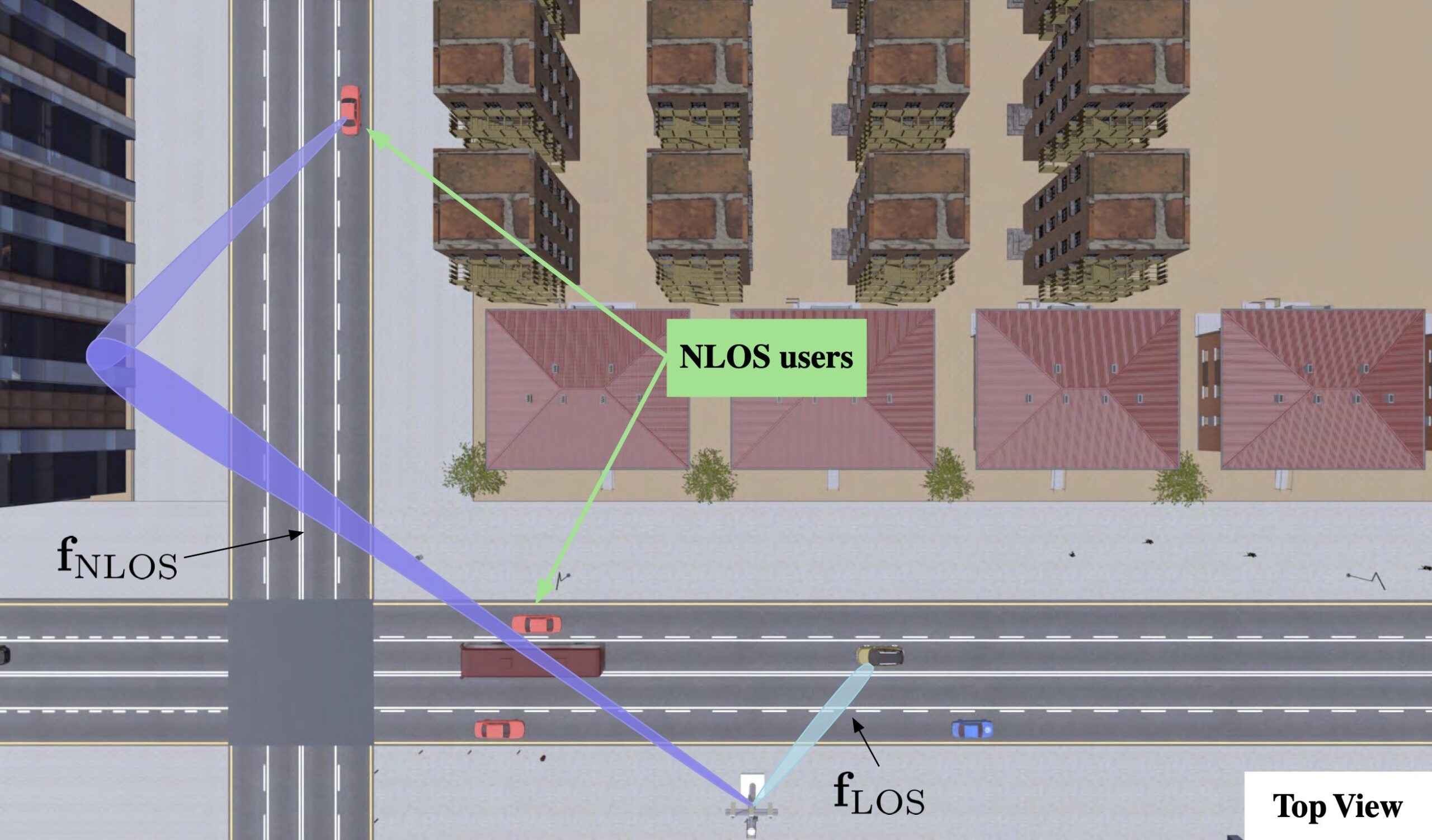
Camera Based mmWave Beam Prediction: Towards Multi-Candidate Real-World Scenarios
Leveraging sensory information to aid the millimeter-wave (mmWave) and sub-terahertz (sub-THz) beam selection process is attracting increasing interest. This sensory data, captured for example by cameras at the basestations, has the potential of significantly reducing the beam sweeping overhead and enabling highly-mobile applications. The solutions developed so far, however, have mainly considered single-candidate scenarios, i.e., scenarios with a single candidate user in the visual scene, and were evaluated using synthetic datasets. To address these limitations, this paper extensively investigates ...... read more
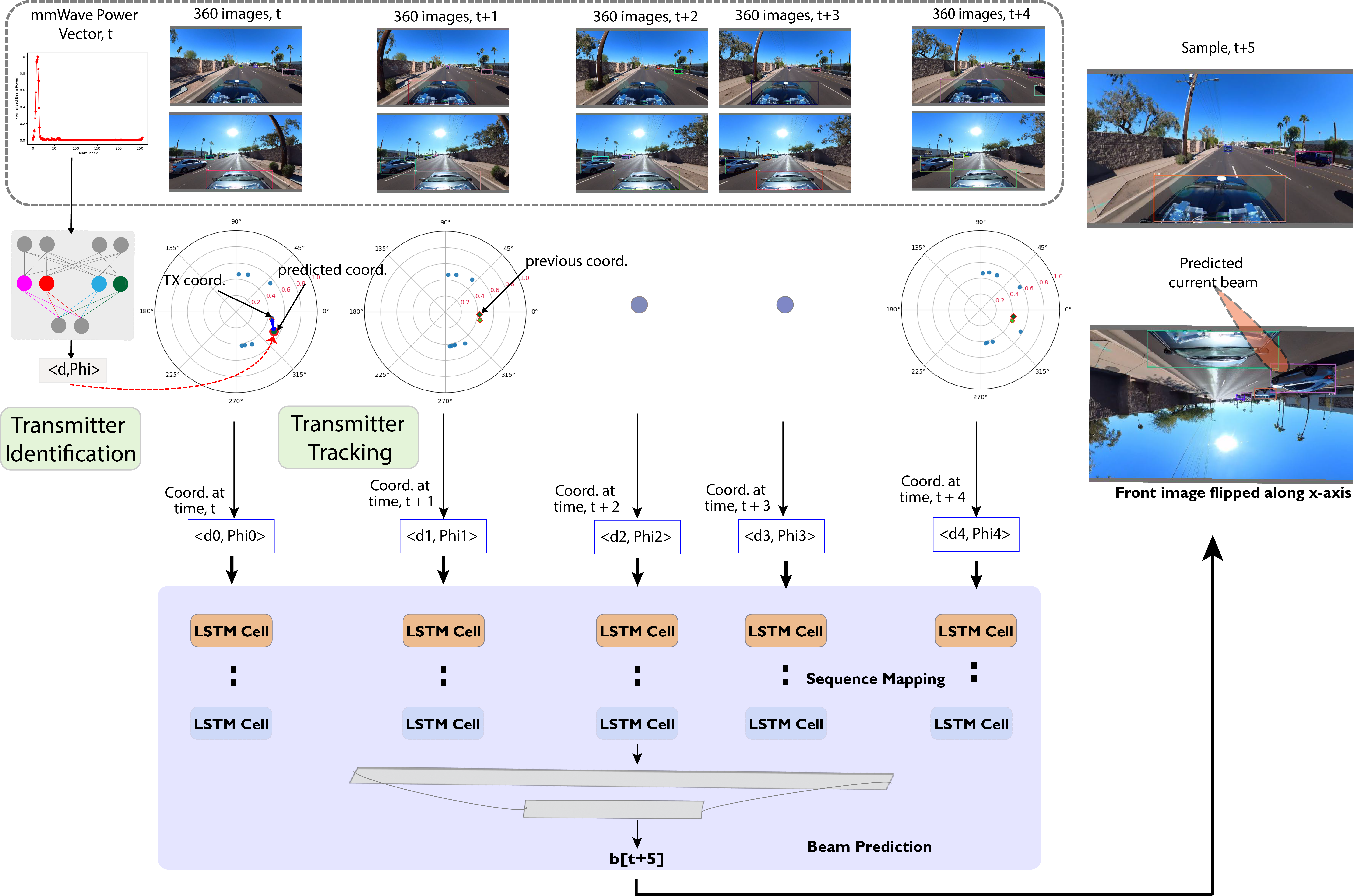
Vehicle Camera Guide mmWave Beams: Approach and Real-world V2V Demonstration
Accurately aligning millimeter-wave (mmWave) and terahertz (THz) narrow beams is essential to satisfy reliability and high data rates of 5G and beyond wireless communication systems. However, achieving this objective is difficult, especially in vehicle-to-vehicle (V2V) communication scenarios, where both transmitter and receiver are constantly mobile. Recently, additional sensing modalities, such as visual sensors, have attracted significant interest due to their capability to provide accurate information about the wireless environment. To that end, in this paper, we develop a deep learning solution.... ... read more
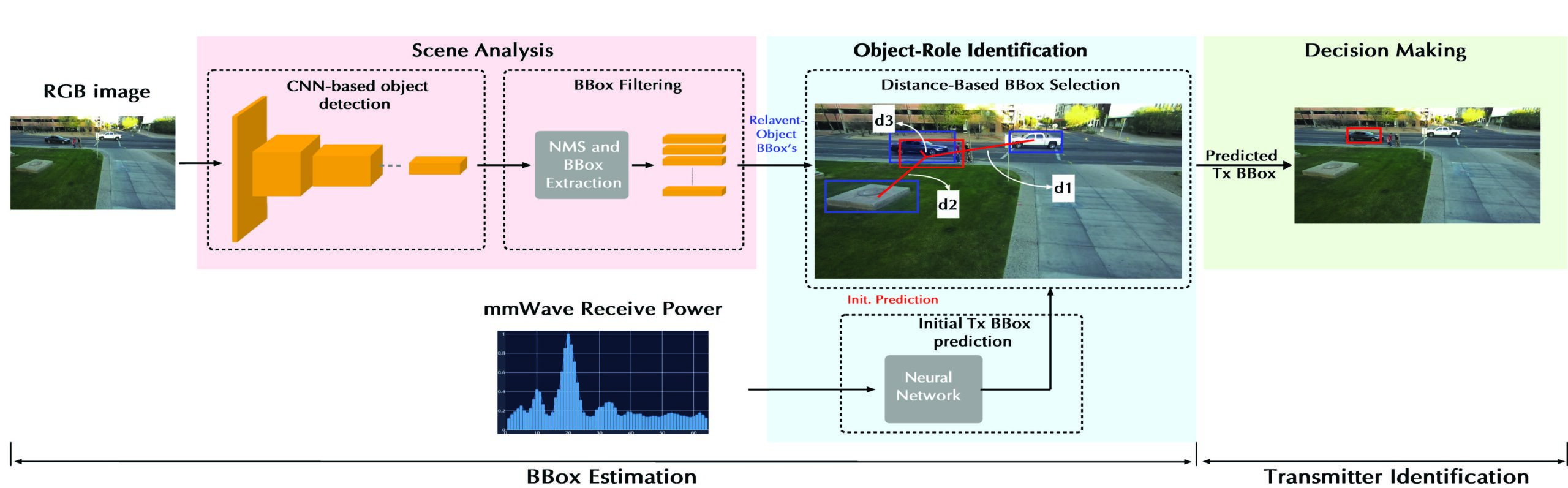
User Identification: The Key Enabler for Multi-User Vision-Aided Wireless Communications
Vision-aided wireless communication is attracting increasing interest and finding new use cases in various wireless communication applications. These vision-aided communication frameworks leverage the visual data captured, for example, by cameras installed at the infrastructure or mobile devices to construct some perception about the communication environment (geometry, users, scatterers, etc.). This is typically achieved through the use of deep learning and advances in computer vision and visual scene understanding. ... read more
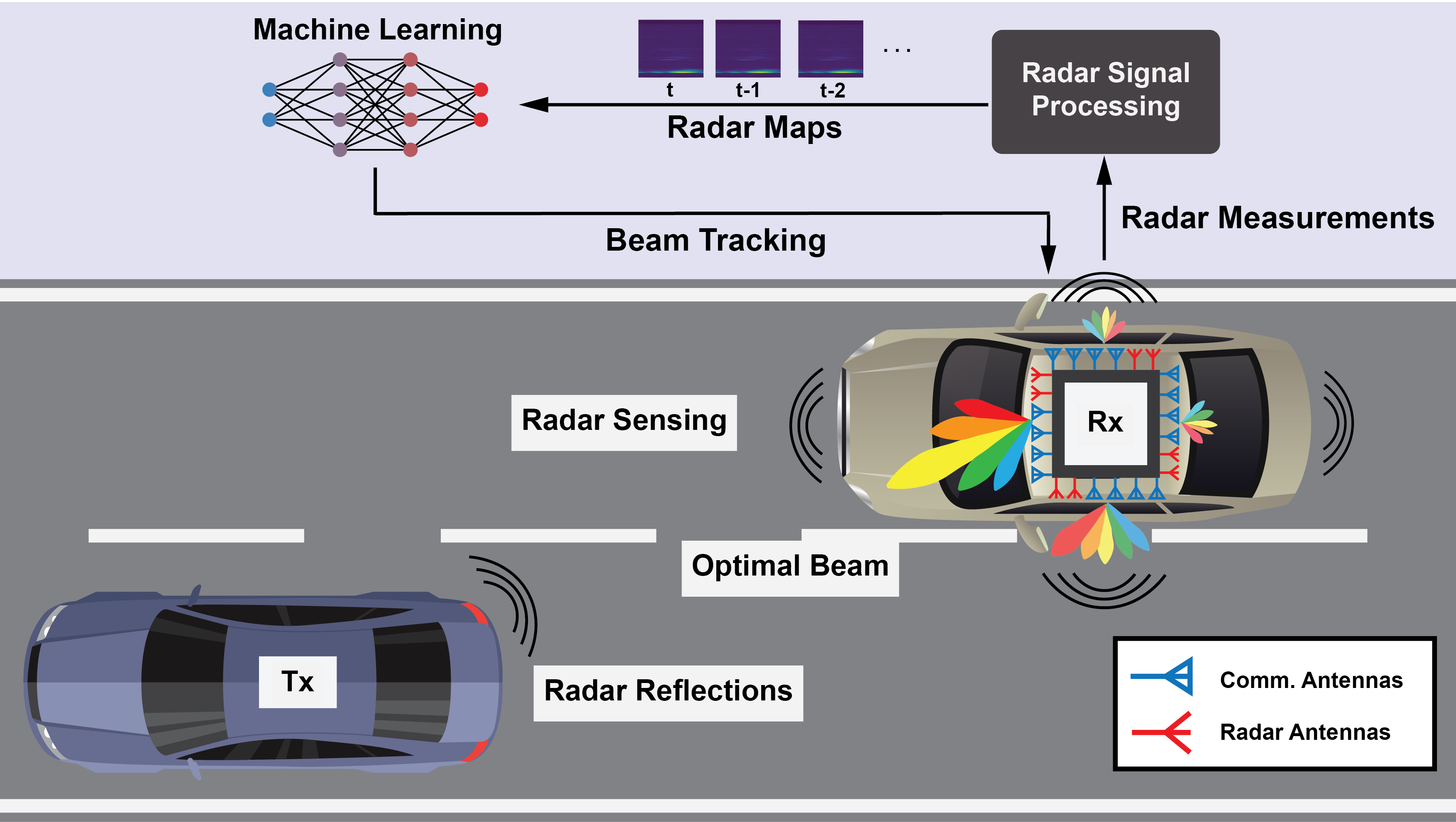
Millimeter Wave V2V Beam Tracking using Radar: Algorithms and Real-World Demonstration
Radar sensing applications for assisting communication have attracted interest thanks to their potential in dynamic environments. A particularly interesting problem for this approach appears in the vehicle-to-vehicle (V2V) millimeter wave and terahertz communication scenarios, where the narrow beams change with the movement of both vehicles. To address this problem ... read more
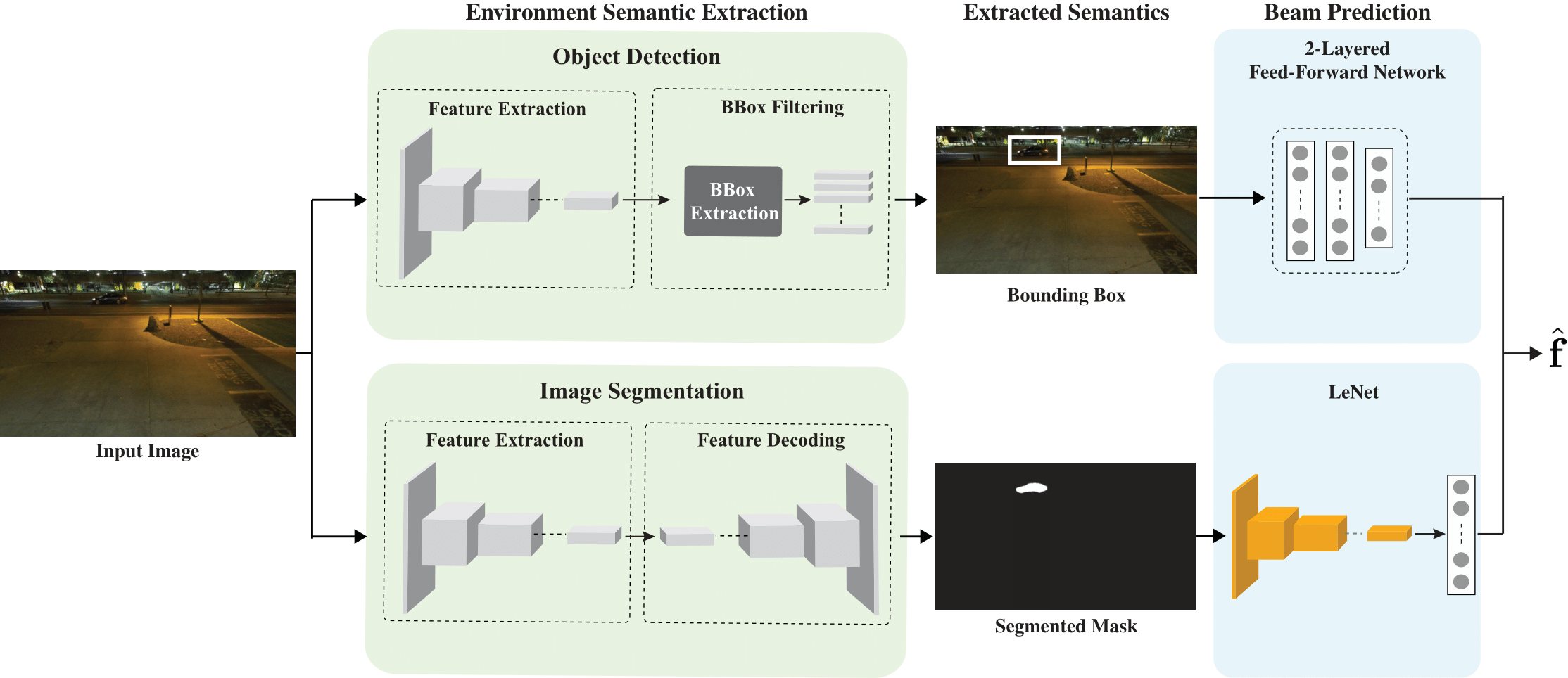
Environment Semantic Aided Communication: A Real World Demonstration for Beam Prediction
Millimeter-wave (mmWave) and terahertz (THz) communication systems adopt large antenna arrays to ensure adequate receive signal power. However, adjusting the narrow beams of these antenna arrays typically incurs high beam training overhead that scales with the number of antennas. Recently proposed vision-aided beam prediction solutions, which utilize raw RGB images captured at the basestation to predict the optimal beams, have shown initial promising results. However, they still have a considerable computational complexity, limiting their adoption in the real world. To address these challenges ... read more
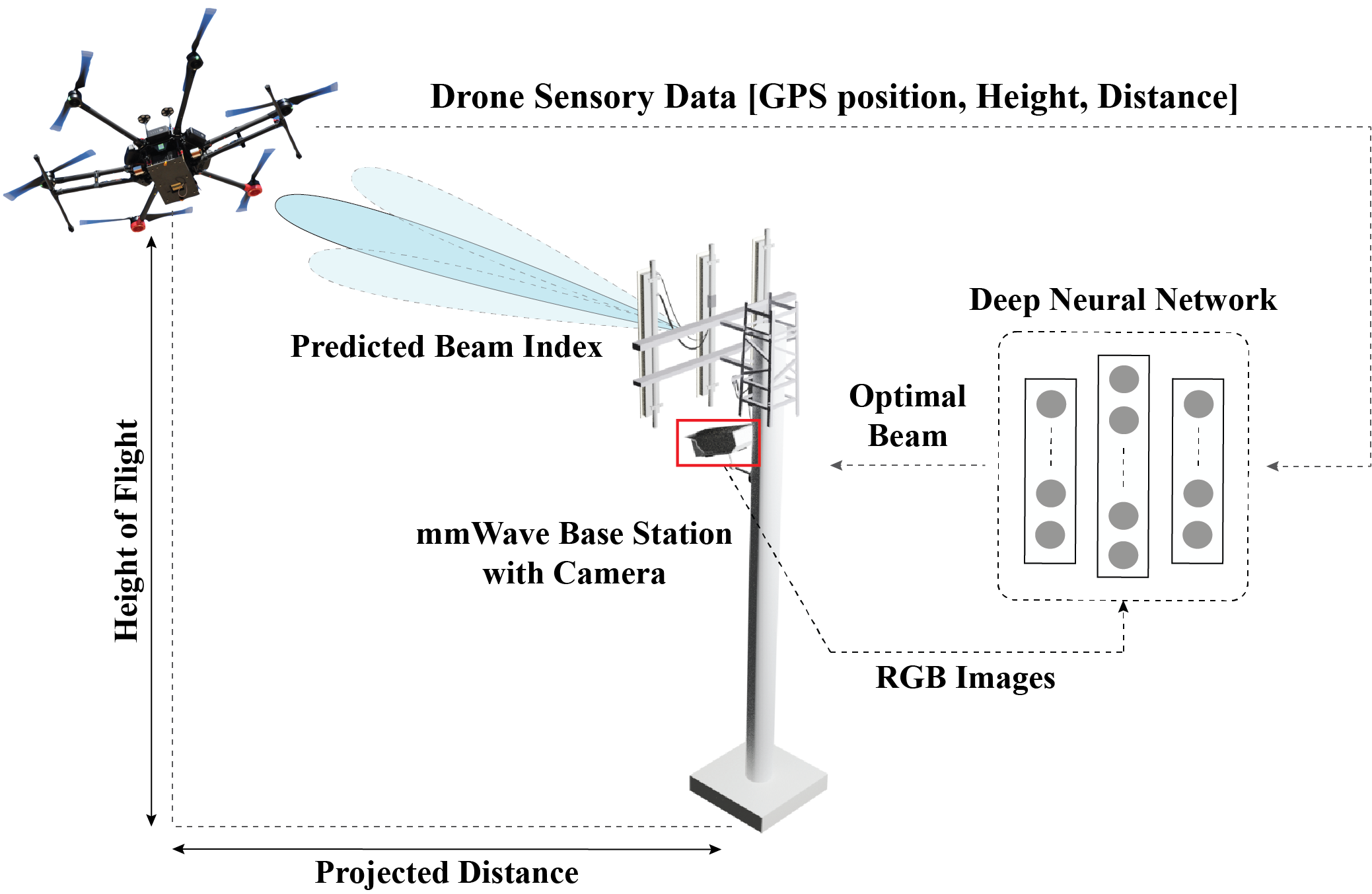
Towards Real-World 6G Drone Communication: Position and Camera Aided Beam Prediction
Millimeter-wave (mmWave) and terahertz (THz) communication systems typically deploy large antenna arrays to guarantee sufficient receive signal power. The beam training overhead associated with these arrays, however, make it hard for these systems to support highly-mobile applications such as drone communication. To overcome this challenge, this paper proposes a machine learning based approach that leverages additional sensory data, such as visual and positional data, for fast and accurate mmWave/THz beam prediction. The developed framework is evaluated on a real-world multi-modal mmWave drone communication dataset comprising co-existing camera, practical GPS, and mmWave beam training data. The proposed sensing-aided solution achieves ... read more
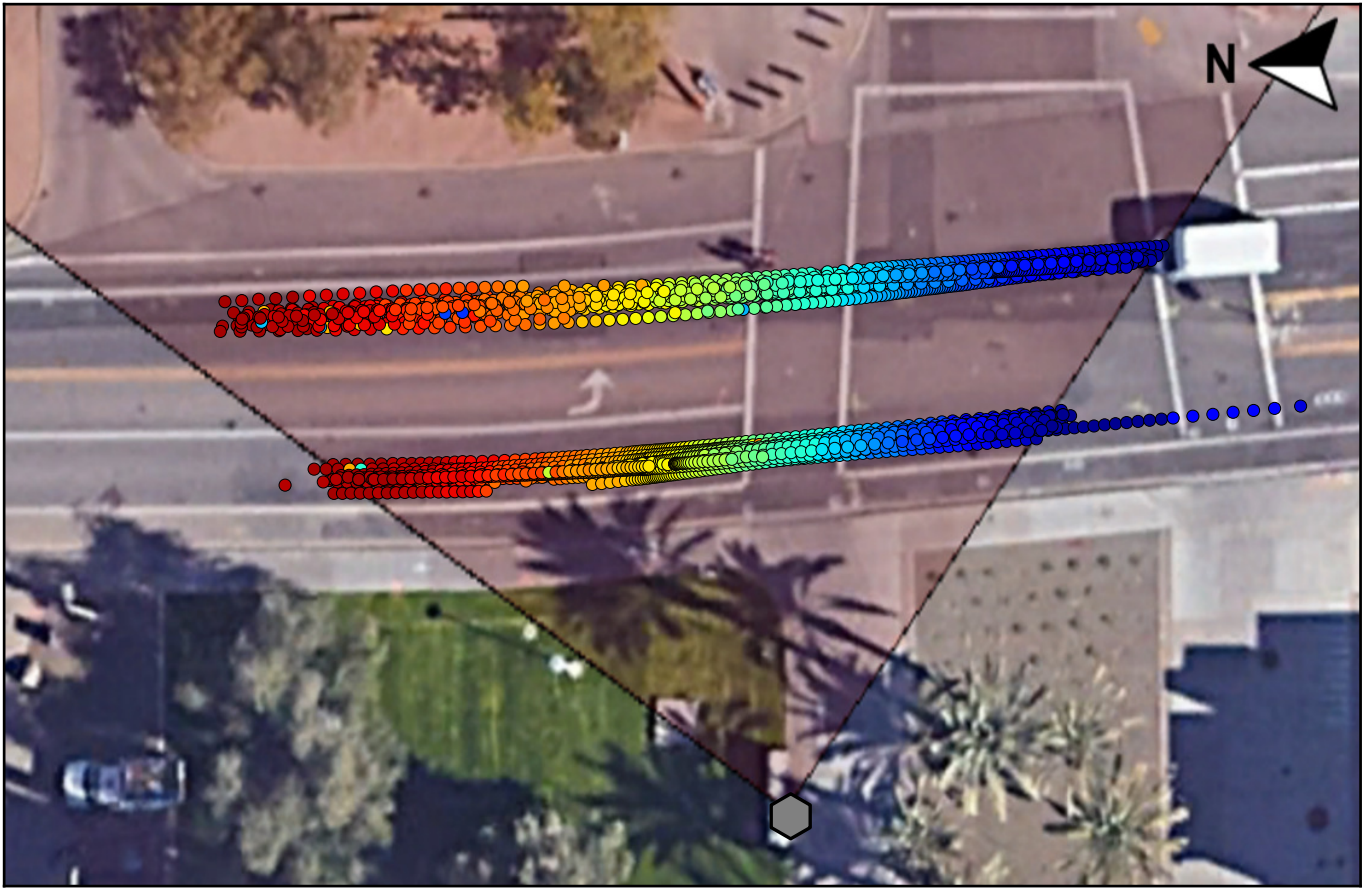
Position-aided Beam Prediction in the Real world: How useful GPS positions actually are?
Millimeter-wave communication systems rely on narrow beams for achieving sufficient receive signal power. Adjusting these beams is typically associated with large training overhead, which becomes particularly critical for highly mobile applications. Intuitively, since optimal beam selection can benefit from the knowledge of the positions of communication terminals, there has been increasing interest in leveraging position data to reduce the overhead in mmWave beam prediction. Prior work, however, studied this problem using only synthetic data that generally does not accurately represent real-world measurements. In this paper, we investigate position-aided beam prediction ... read more
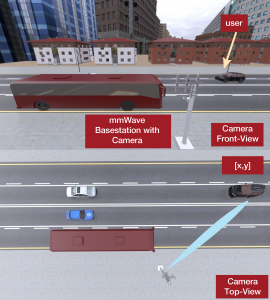
Vision-Position Multi-Modal Beam Prediction Using Real mmWave Datasets
One of the significant challenges associated with mmWave/THz communication systems is the large beam training overhead, especially for high-mobility scenarios. The interesting question here is, can additional sensory data such as data captured from the position and visual (RGB camera) sensors be utilized to reduce the beam training overhead and accurately predict the mmWave beams in 5G/B5G systems? This work attempts to answer this question using a large real-world diverse (different locations, scenarios, etc.) dataset in Vehicle-to-Infrastructure (V2I) mmWave communication scenarios. ... read more
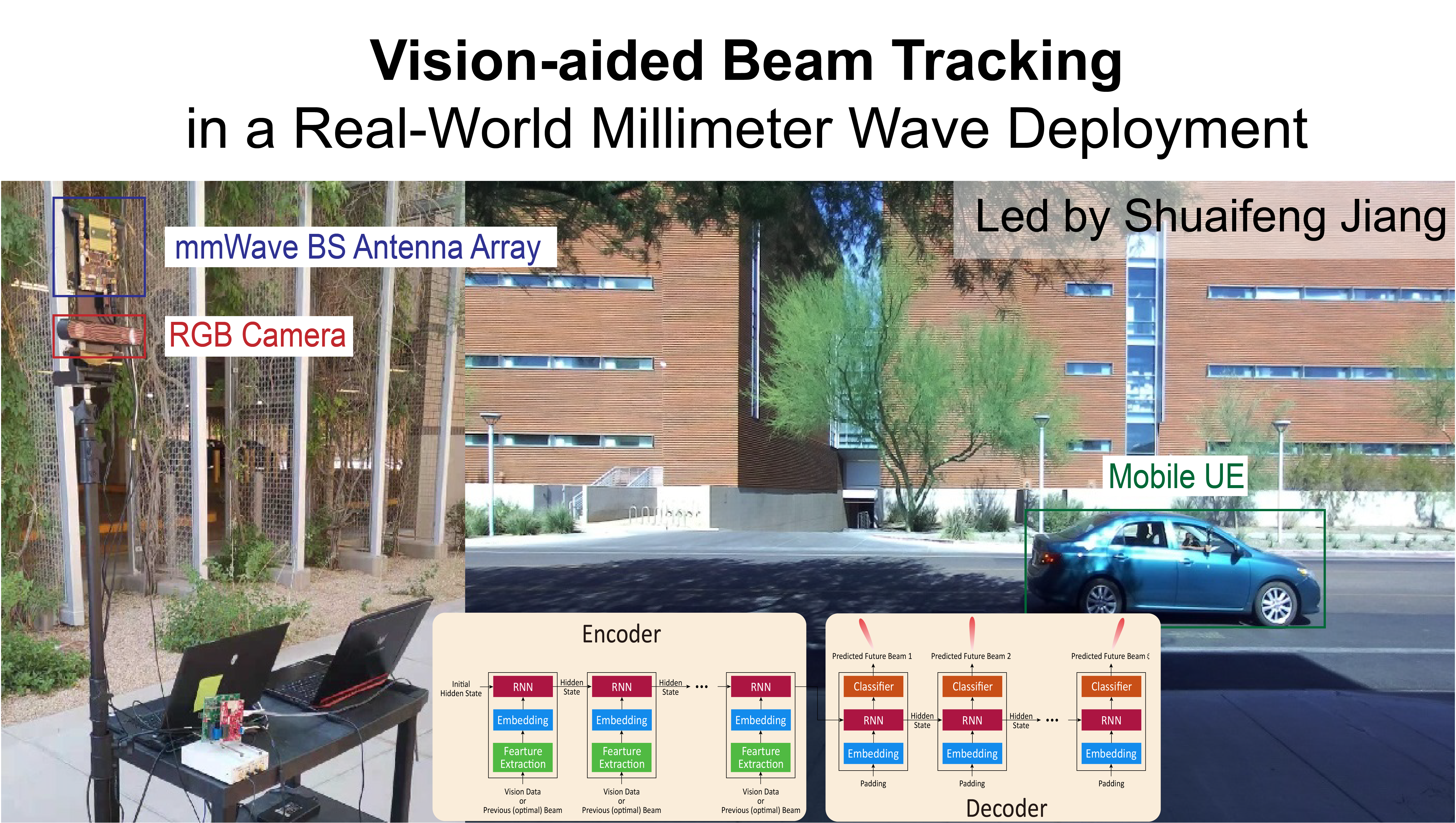
Computer Vision Aided Beam Tracking in A Real-World Millimeter Wave Deployment
This paper utilizes visual sensing information to enable fast and low-overhead mmWave/THz beam tracking. In this paper, we first propose a universal problem formulation for the auxiliary data-aided beam tracking, which could be used for different auxiliary data modalities. Then we propose a deep learning model for vision-aided mmWave/THz beam tracking exploiting an encoder-decoder architecture. The proposed vision-aided beam tracking is evaluated on the DeepSense real-world dataset ... read more
Radar Aided 6G Beam Prediction: DL Algorithms and Real-World Demonstration
This paper presents the first machine learning based real-world demonstration for radar-aided beam prediction in a practical vehicular communication scenario. Leveraging radar sensory data at the communication terminals provides important awareness about the transmitter/receiver locations and the surrounding environment, which is leveraged for selecting the beams ... read more
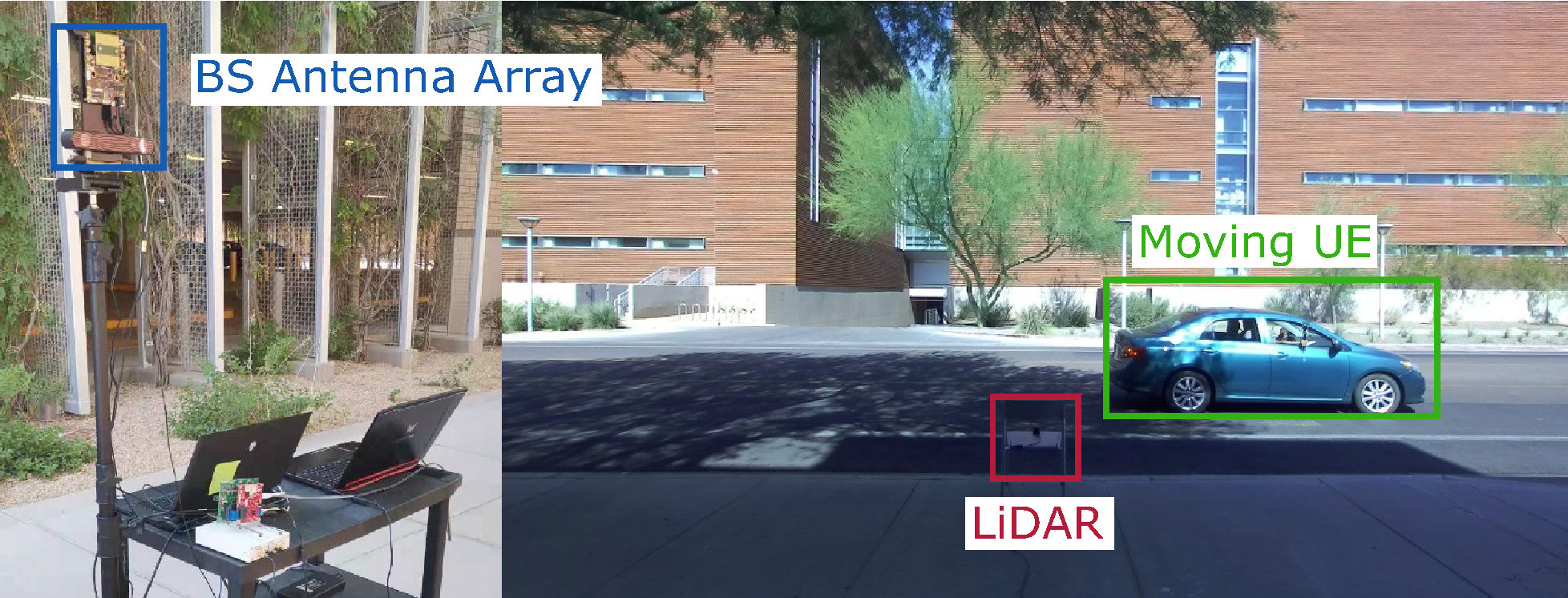
LiDAR Aided Future Beam Prediction in Real-World Millimeter Wave V2I Communications
This paper presents the first large-scale real-world evaluation for using LiDAR data to guide the mmWave beam prediction task. A machine learning (ML) model that leverages the LiDAR sensory data to predict the current and future beams was developed. Based on the large-scale real-world dataset, DeepSense 6G, this model was evaluated in a vehicle-to-infrastructure communication scenario with highly-mobile vehicles ... read more
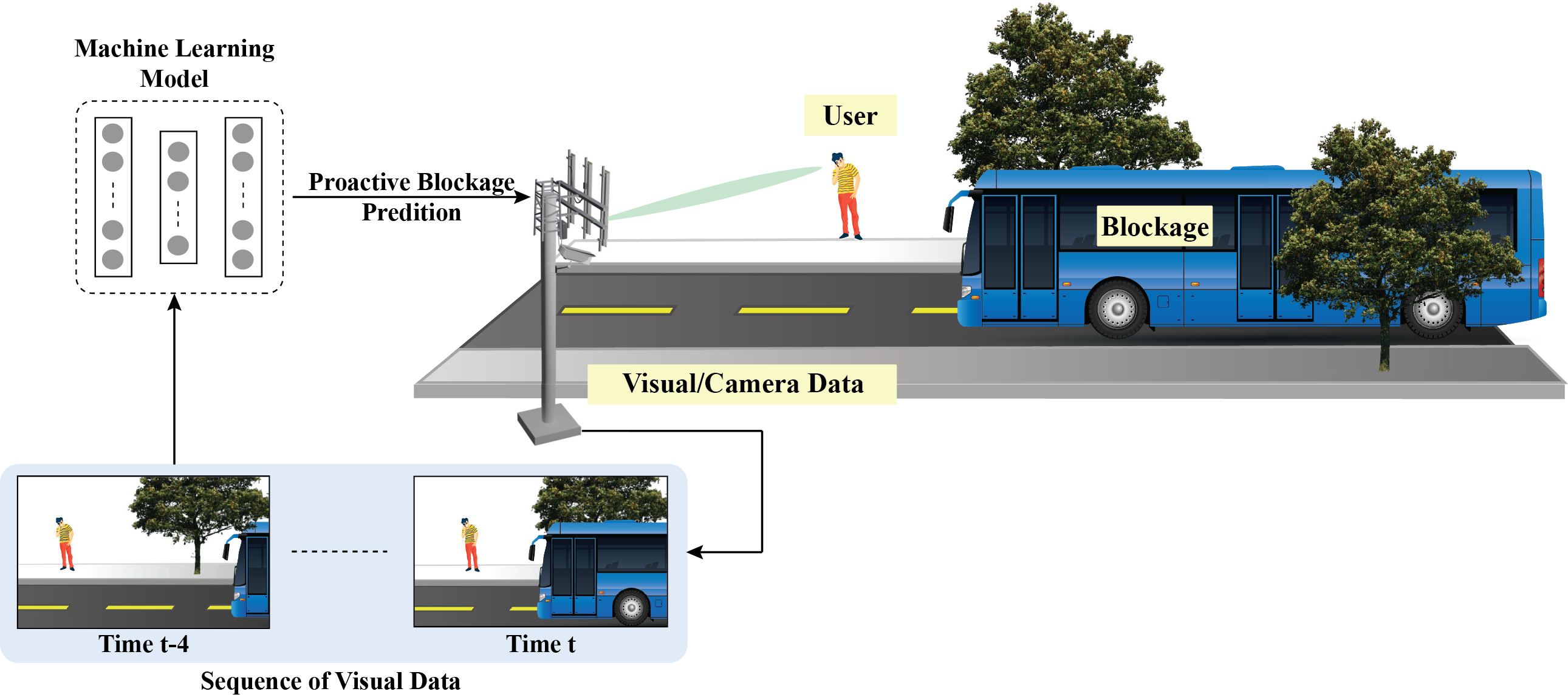
Computer Vision Aided Blockage Prediction in Real-World Millimeter Wave Deployments
This paper provides the first real-world evaluation of using visual (RGB camera) data and machine learning for proactively predicting millimeter wave (mmWave) dynamic link blockages before they happen. Proactively predicting line-of-sight (LOS) link blockages enables mmWave/sub-THz networks to make proactive network management decisions, such as proactive beam switching and hand-off) before a link failure happens. This can significantly enhance the network reliability and latency while efficiently utilizing the wireless resources. To evaluate this gain in reality, this paper ... read more
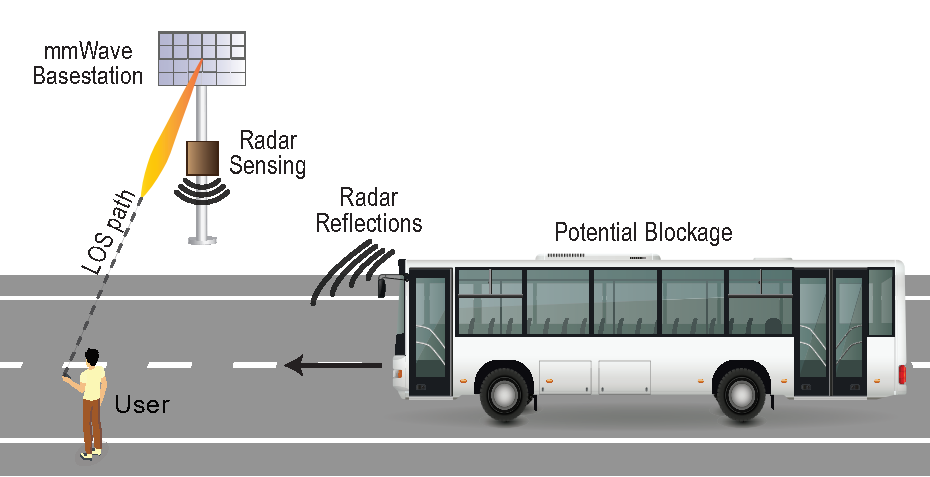
Radar Aided Proactive Blockage Prediction in Real-World Millimeter Wave Systems
In this paper, we propose to utilize radar sensors to
provide sensing information about the surrounding environment
and moving objects, and leverage this information to proactively
predict future link blockages before they happen ... read more
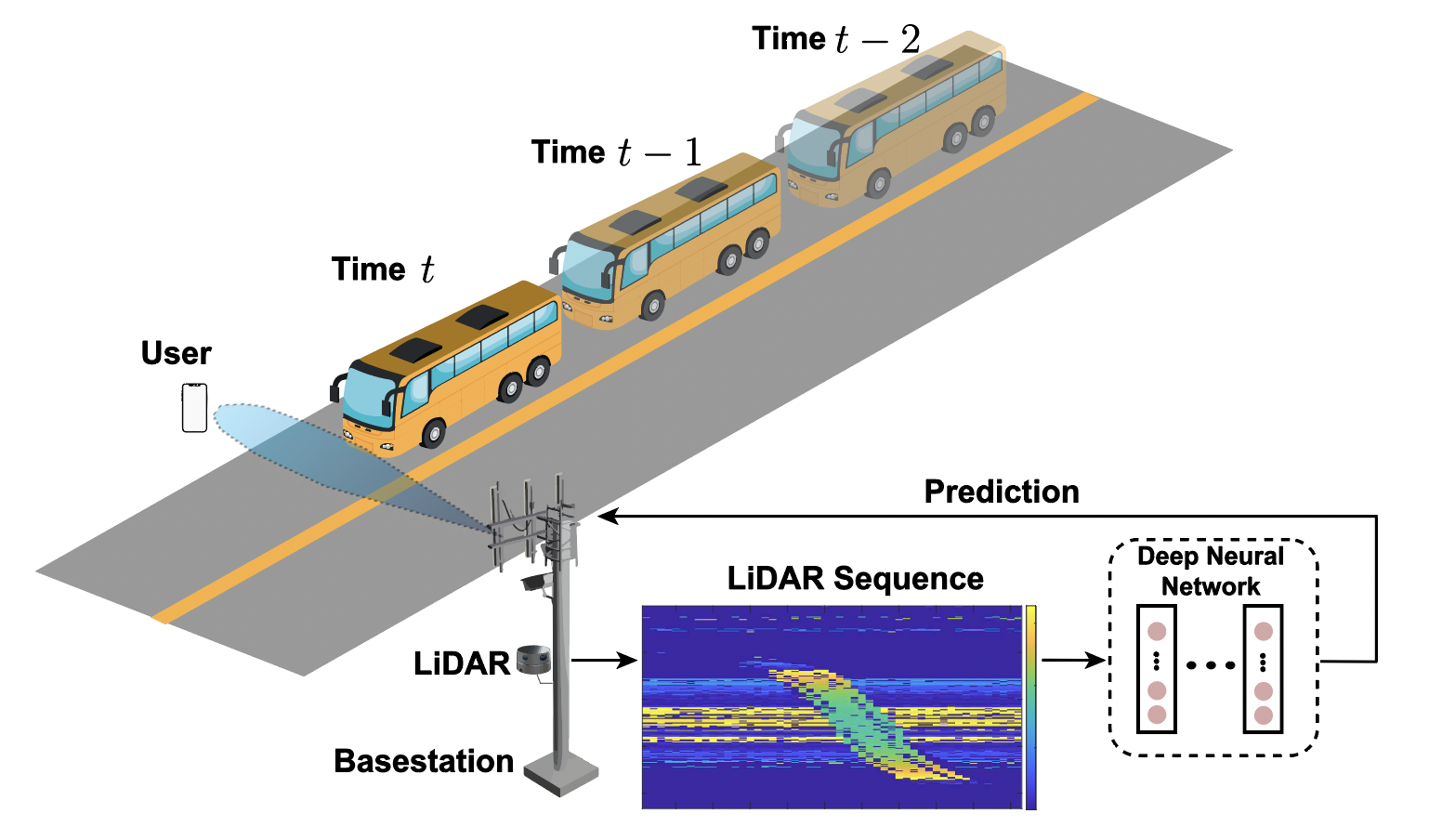
LiDAR-Aided Mobile Blockage Prediction in Real-World Millimeter Wave Systems
A typical LiDAR sensor sends pulsed light waves into the surrounding environment. These pulses are reflected by the objects and returned to the sensor; and the sensor uses the round-trip time to calculate the distance it traveled. By sending and receiving laser beams, the LiDAR sensor collects a 2-D point cloud map of the surrounding environment. We propose to leverage these LiDAR sensory data to detect if an object is going to block the mmWave communication link between the basestation and the mobile user. This figure shows an example when a communication link is getting blocked by a moving object ... read more
Blockage Prediction Using Wireless Signatures in a Real-World Demonstration
The blockage prediction approaches in this paper are based on the following simple observation: Consider a fixed TX and RX in a certain environment with a LOS path. If an object moves in this environment till it blocks this LOS path, then, during the movement, the object acts like a scatterer for the signal propagating from the TX to the RX. The received signal during this interval will experience a constructive and destructive interference from the LOS ray and the ray scattered on the moving object. ... read more