DeepSense ML Challenges
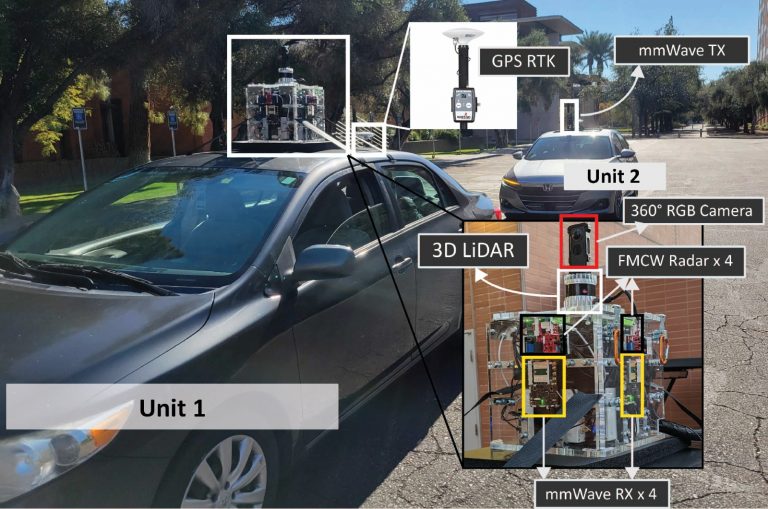
DeepSense-ITU Multi Modal V2V Beam Tracking Challenge 2023
Vehicle-to-vehicle (V2V) communication is indispensable for intelligent transportation systems (ITS) to enable crucial data exchange among vehicles, enhancing safety, traffic efficiency, and driving experience. Yet, conventional V2V methods struggle with the escalating data complexity, prompting exploration of higher frequencies like millimeter wave (mmWave/sub-THz). However, this shift presents challenges like deploying large antenna arrays and using narrow directive beams to ensure sufficient receive power, resulting in significant beam training overhead. This challenge presents a critical bottleneck for high-mobility, latency-sensitive V2V applications, paving the way for the next V2V frontier: efficient and effective beam prediction/tracking.
Given a multi-modal training dataset comprising sequences of 360-degree RGB images and vehicle positions from different locations with diverse environmental characteristics, the goal is to develop machine learning models capable of accurate sensing-aided V2V beam tracking.
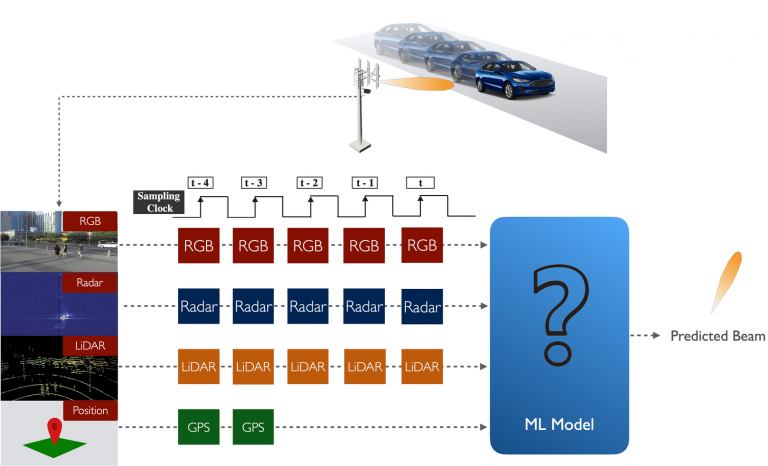
DeepSense-ITU Multi Modal Beam Prediction Challenge 2022
The mmWave systems in 5G and potentially sub-terahertz in 6G and beyond) require deploying large antenna arrays at the transmitters and/or receivers and using narrow beams to guarantee sufficient receiver power. Finding the best beams (out of a pre-defined codebook) at the transmitter and receiver is associated with high beam training overhead (search time to find/align the best beams), which makes it difficult for these systems to support highly-mobile and latency-sensitive applications.
Given a multi-modal training dataset consisting of data collected at different locations with diverse environmental features, the objective is to develop machine learning-based models that can adapt to and perform accurate sensing-aided beam prediction at an entirely new location (not part of the training dataset). For more details on this ML challenge, please click on the link below.
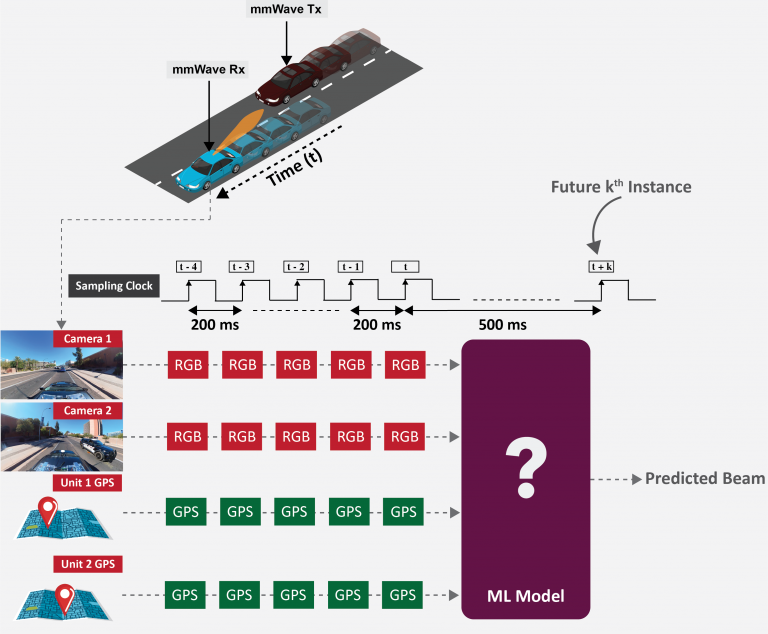
DeepSense-ITU Multi Modal V2V Beam Prediction Challenge 2023
V2V communication is crucial in intelligent transportation systems, enabling vehicles to exchange information for improved safety, traffic efficiency, and driving experience. However, traditional methods face difficulty in handling the increasing data volume and complexity, limiting the effectiveness of ITS. Exploring higher frequency bands like millimeter wave and sub-terahertz frequencies is important to overcome this. These bands offer larger bandwidths, addressing the growing data rate demands of V2V communication. However, deploying large antenna arrays and using narrow beams in high-frequency systems introduces challenges, particularly in beam training overhead. Overcoming this challenge is crucial for effective and efficient V2V beam prediction, particularly for high-mobility and latency-sensitive V2V applications.
Given a multi-modal training dataset comprising sequences of 360-degree RGB images and vehicle positions from different locations with diverse environmental characteristics, the goal is to design machine learning models capable of accurate sensing-aided V2V beam tracking.