Scenario 30
License
Overview
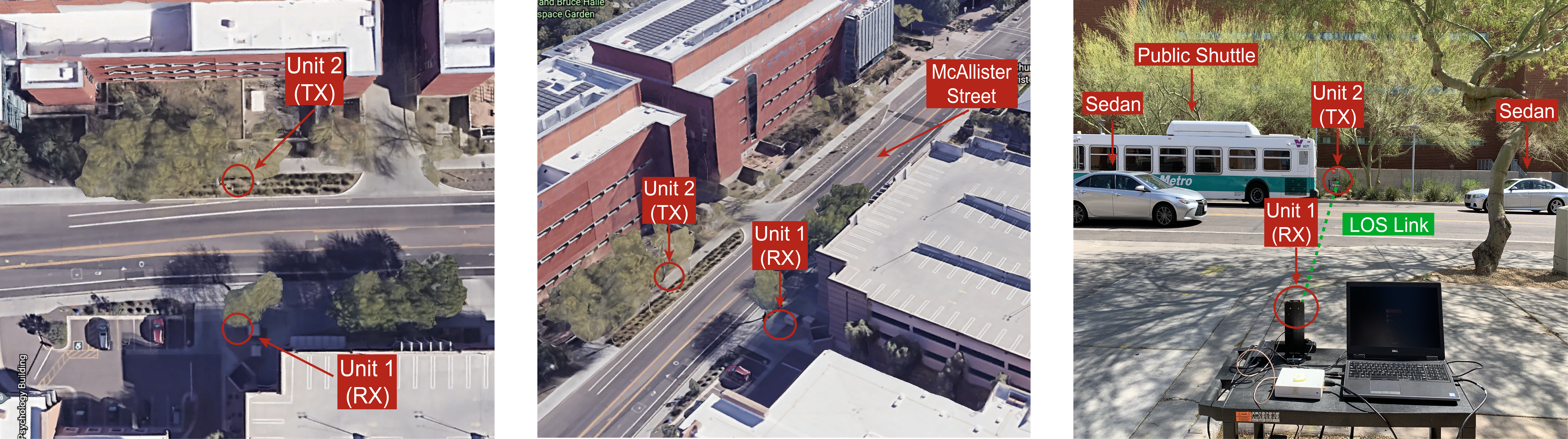
The data collection setup of Scenarios 30 (outdoor). The left subfigure shows the exact locations of Unit 1 and Unit 2; the middle subfigure shows the full view of the street and surroundings; the right subfigure shows the traffic and street view from Unit 1 perspective.
Scenario 30 is collected in an outdoor wireless environment representing a two-way city street. The DeepSense Testbed 3 is deployed in this environment where the two units are placed on the opposite sides of the street. The transmitter (Unit 2) constantly transmits using one antenna element of the phased array to realize omnidirectional transmission. The receiver continuously scans the surrounding environment using a receive mmWave beam steering codebook of 64 beams and by measuring the receive power with each beam. The testbed collects data samples at a dynamic rate ranging from 6 to 12 samples/s. The average sampling rate is 9 samples/s. Each data sample includes an RGB image and a 64-element receive power vector, both collected by Unit 1. Please refer to the detailed description of the testbed presented here.
McAllister Ave: It is a two-way street with 2 lanes, a width of 10.6 meters, and a vehicle speed limit of 25mph (40.6 km per hour. It is worth mentioning here that since this is a city street, vehicles of various sizes and travel speeds pass in both directions. This creates a diverse pool of blockages for the LOS link between the transmitter and receiver, which results in diverse received power maps (i.e., diverse power fluctuations across all 64 beam over time instances) and a diverse dataset.
Scenarios 17-22 are outdoor wireless environments representing a two-way city street. The DeepSense Testbed 3 is deployed in this environment where the two units are placed on the opposite sides of the street. The transmitter (Unit 2) constantly transmits using one antenna element of the phased array to realize omnidirectional transmission. The receiver continuously scans the surrounding environment using a receive mmWave beam steering codebook of 64 beams and by measuring the receive power with each beam. The testbed collects data samples at a dynamic rate ranging from 6 to 12 samples/s. The average sampling rate is 10 samples/s. Each data sample includes an RGB image and a 64-element receive power vector, both collected by Unit 1. Please refer to the detailed description of the testbed presented here.
McAllister Ave: It is a two-way street with 2 lanes, a width of 10.6 meters, and a vehicle speed limit of 25mph (40.6 km per hour. It is worth mentioning here that since this is a city street, vehicles of various sizes and travel speeds pass in both directions. This creates a diverse pool of blockages for the LOS link between the transmitter and receiver, which results in diverse received power maps (i.e., diverse power fluctuations across all 64 beam over time instances) and a diverse dataset.
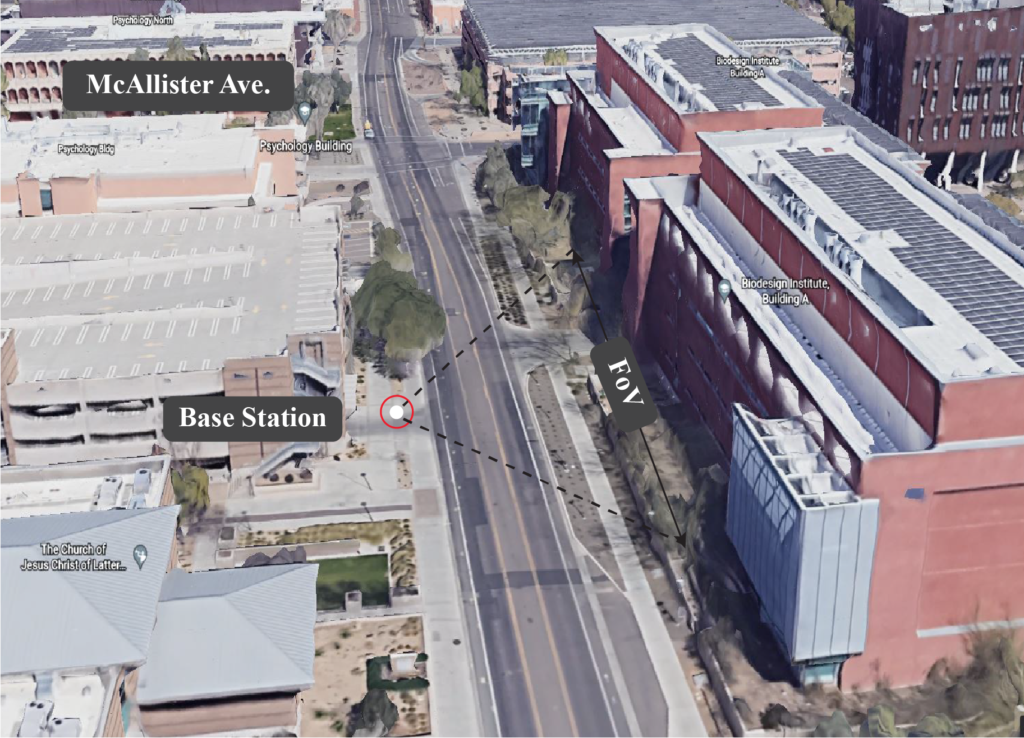
Collected Data
Overview
Number of Data Collection Units: 2 (using DeepSense Testbed #3)
Total Number of Data Samples: 760000
Data Modalities: RGB images, 64-dimensional received power vector, GPS locations
Sensors at Unit 1: (Stationary Receiver)
- Wireless Sensor [Phased Array]: A 16-element antenna array operating in the 60 GHz frequency band and receives the transmitted signal using an over-sampled codebook of 64 pre-defined beams
- Visual Sensor [Camera]: The main visual perception element in the testbed is an RGB-D camera. The camera is used to capture RGB images of 960×540 resolution at a base frame rate of 30 frames per second (fps)
- Position Sensor [GPS Receiver]: A GPS-RTK receiver for capturing accurate real-time locations for the stationary unit 1
- LiDAR Sensor [2D laser scanner]: This system provides the range and angle data of objects within its range of view. This system covers a maximum range of 16 meters around the master component and it operates at a maximum scan rate of 10 Hz.
- MmWave radar sensor [Ranging sensor]: For the Frequency Modulated Continuous Wave (FMCW) radar, we adopted a set of radar parameters based on the Texas Instrument (TI’s) short range radar (SRR) example, given by B = 750 MHz, µ = 15 MHz/us, A = 128 chirps/frame, S = 256 samples/chirp. These settings provide the maximum range of 45m and the maximum velocity of 56 km/h.
- MmWave radar sensor [Ranging sensor]: For the Frequency Modulated Continuous Wave (FMCW) radar each sample contains a 3D complex radar frame samples of (# of RX antennas) x (# of samples per chirp) x (# of chirps per frame).
- Active TX antennas: 1
- Active RX antennas: 4
- # of samples per chirp: 256
- # of chirps per frame: 128
- ADC sampling rate: 5000 Ksps
- Chirp slope: 15.015 MHz/us
- Chirp Start Frequency: 77 GHz
- Ramp end time: 60 us
- ADC start time: 6 us
- Idle time: 5 us
- Receiver gain: 30dB
- Radar frames per second: 10
Sensors at Unit 2: (Mobile Transmitter)
- Wireless Sensor [Phased Array]: A stationary 60 GHz mmWave transmitter. The transmitter (Unit 2) constantly transmits using one antenna element of the phased array to realize omnidirectional transmission
- Position Sensor [GPS Receiver]: A GPS-RTK receiver for capturing accurate real-time locations for the stationary unit 2
Testbed | 3 |
---|---|
Instances | 76000 |
Number of Units | 2 |
Data Modalities | RGB images, 64-dimensional received power vector, GPS locations, LiDAR point cloud, radar data |
Unit1 | |
Type | Stationary |
Hardware Elements | RGB camera, mmWave phased array receiver, GPS receiver, , LIDAR and mmWave radar |
Data Modalities | RGB images, 64-dimensional received power vector, GPS locations, LiDAR point cloud, radar data |
Unit2 | |
Type | Stationary |
Hardware Elements | mmWave omni-directional transmitter, GPS receiver |
Data Modalities | GPS locations |
Data Visualization
Download
Please login to download the DeepSense datasets
How to Access Scenario X Data?
Step 1. Download Scenario Data
Step 2. Extract the scenario30.zip file
Scenario X folder consists of three sub-folders:
- unit1: Includes the data captured by unit 1
- unit2: Includes the data captured by unit 2
- resources: Includes the scenario-specific annotated dataset, data labels, and other additional information. For more details, refer to the resources section below.
The scenario X folder also includes the “scenario 30.csv” file with the paths to all the collected data. For each coherent time, we provide the corresponding visual, wireless, and GPS data.
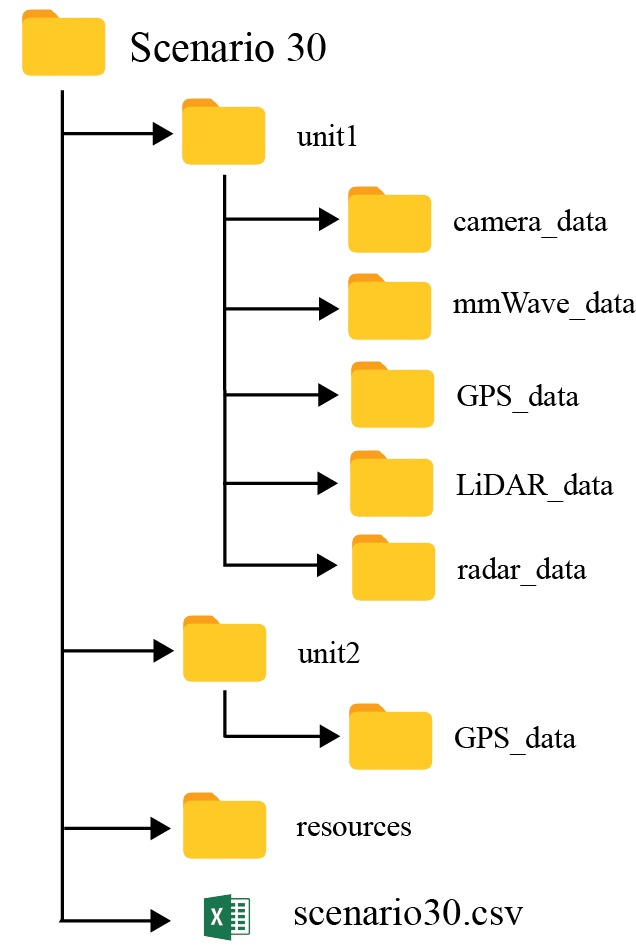
Resources
What are the Additional Resources?
Resources consist of the following information:
- data labels: The labels comprises of the ground-truth link blockage status, where ‘0’ represent line-of-sight (LOS) connection and ‘1’ represent blocked connection
- additional information: Includes the scenario-specific additional data. Details of the information is provided below
Data Labels
The label comprises of the ground-truth link-status manually annotated from the RGB images
- Ground-Truth Blockage: We utilize the RGB images and manually annotate the data samples with link-status labels. More specifically, a link-status label of ‘1’ is assigned to a time instance when the LOS link is blocked and a label of ‘0’ otherwise.
Additional Information
We, further, provide additional information for each sample present in the scenario dataset. The contents of the additional data is listed below:
- index: It represents the sample number
- time_stamp[UTC]: This represents the time of data capture in “hr-mins-secs-ms” format
Warning on NaN values: Some samples (<1%) in the column ‘unit1_pwr_60ghz’ contain NaNs (not-a-value). These NaNs result from random, real-world sensor errors. In the context of received power, NaNs can be considered as zeros. Assuming that pwr_vect
is our received power vector, one can explicitly convert the NaNs into zeros by doing pwr_vect(isnan(
pwr_vect)) = 0
in Matlab or pwr_vect[np.isnan(
pwr_vect)] = 0
in Python.